In the global race to combat climate change, solar technology is undergoing a transformative revolution. Perovskite materials, hailed as the “next-generation photovoltaic stars,” are gaining prominence for their exceptional optoelectronic properties. Not only do they theoretically surpass the efficiency limits of traditional silicon-based solar cells, but their production energy consumption is a mere tenth of conventional materials. This thin-film technology enables the creation of translucent or flexible modules, unlocking novel applications such as building-integrated photovoltaics (BIPV) and wearable device power supplies.
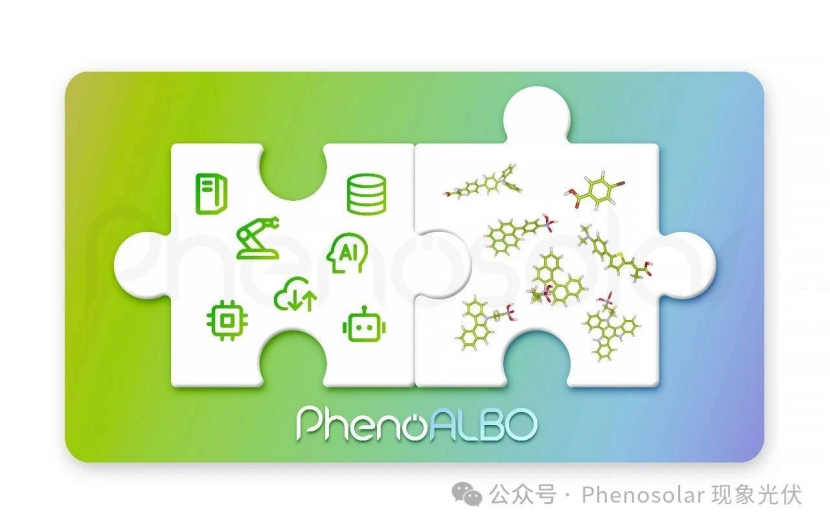
At the heart of this perovskite photovoltaic (PV) revolution lies materials innovation, with self-assembled monolayers (SAMs) emerging as a critical interface modifier. SAMs directly dictate energy-level alignment and device stability in hole-transport layers (HTLs). However, traditional materials R&D faces a “needle-in-a-haystack” challenge: scientists must screen ideal molecules from combinatorial possibilities exceeding Earth’s sand grains, a process often spanning years. Dubbed the “trial-and-error dilemma,” this bottleneck has now been addressed by PhenoALBO (Active Learning + Bayesian Optimization), an intelligent materials development system described as a “molecular microscope” for researchers. Leveraging machine learning predictions, quantum chemistry simulations, and robotic experimentation, PhenoALBO elevates materials R&D efficiency to unprecedented levels.
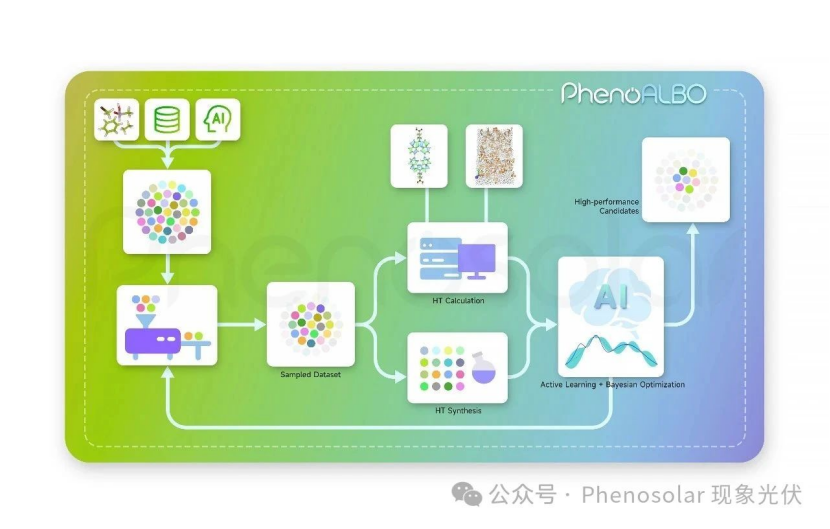
PhenoALBO establishes a closed-loop workflow for SAMs molecular engineering, comprising four core modules: an intelligent molecular design engine, high-throughput computational simulations, high-throughput molecular synthesis, and an AI-driven optimization/screening system. This framework transitions R&D from manual trial-and-error to AI-driven precision.
The intelligent molecular design engine employs three primary pathways for new SAMs generation:
- Leveraging PhenoALBO’s extensive SAMs laboratory experience and structural insights to craft bespoke molecules.
- Mining literature and organic molecule databases for high-potential candidates.
- Utilizing transfer learning-based generative models to synthesize novel SAMs-compliant molecular structures.
Post-algorithm screening, candidates undergo high-throughput density functional theory (DFT) calculations (to extract HOMO/LUMO quantum properties), molecular descriptor analysis (e.g., molecular weight, atomic composition), and molecular dynamics simulations of thin-film self-assembly. Leading candidates are synthesized and experimentally validated for photovoltaic performance metrics like power conversion efficiency (PCE) and open-circuit voltage (Vₒc). Combined with active learning AI and Bayesian optimization, this data-driven loop iteratively refines material sampling and screening, optimizing multi-objective performance criteria to yield high-efficiency SAMs functional molecules.
This integrated molecular generation-theoretical prediction-experimental validation closed-loop system dramatically expands the design space and accelerates R&D timelines, positioning perovskite-SAMs technology as a cornerstone of sustainable energy innovation.